Biotech and AI is rapidly transforming healthcare, offering innovative solutions for drug discovery, personalized medicine, and research. This intersection of biological sciences and artificial intelligence presents both exciting possibilities and significant challenges. From accelerating drug development to analyzing vast biological datasets, the convergence of biotech and AI promises to reshape the future of medicine and beyond.
The integration of these two fields is not just theoretical; concrete applications are already emerging. AI algorithms are being utilized to identify potential drug candidates more efficiently, predict patient responses to therapies, and even develop personalized treatments. This has the potential to dramatically improve patient outcomes and accelerate scientific breakthroughs.
Introduction to Biotech and AI Convergence
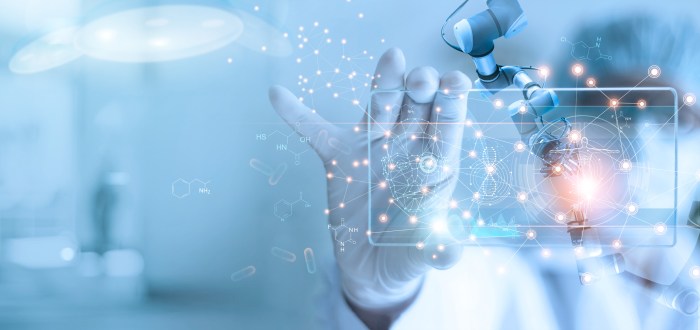
The convergence of biotechnology and artificial intelligence (AI) is rapidly reshaping scientific discovery and technological innovation. This intersection offers unprecedented opportunities to accelerate progress in various fields, from drug development to personalized medicine. However, careful consideration of ethical implications and potential challenges is essential to ensure responsible advancement.The integration of AI algorithms with biological data allows for more sophisticated analysis and interpretation, enabling researchers to identify patterns and correlations that might otherwise remain hidden.
This enhanced understanding promises to accelerate the pace of scientific breakthroughs, ultimately leading to improved human health and welfare. Simultaneously, the need for robust data management and ethical considerations surrounding AI applications in healthcare must be addressed to maintain public trust and ensure responsible innovation.
Applications of AI in Biotechnological Research
AI is transforming various aspects of biotechnological research, from accelerating drug discovery to improving diagnostics. The ability to analyze massive datasets, identify patterns, and predict outcomes is revolutionizing the way we approach biological problems.
- Drug Discovery and Development: AI algorithms can analyze vast amounts of chemical and biological data to identify potential drug candidates. This approach accelerates the identification process, reducing the time and cost associated with traditional methods. For example, AI-powered platforms are used to screen thousands of compounds to identify those with high probability of binding to specific molecular targets, significantly speeding up the lead identification phase in drug discovery.
- Personalized Medicine: AI can analyze patient data, including genetic information, lifestyle choices, and medical history, to tailor treatment plans. This approach allows for personalized medicine that is optimized for individual patient needs, resulting in more effective therapies and improved health outcomes. For instance, AI can predict the likelihood of a patient developing a particular disease based on their genetic profile and lifestyle, allowing for preventative measures to be taken in advance.
- Genomics and Bioinformatics: AI algorithms can analyze complex genomic data to identify disease-causing mutations and understand gene function. This knowledge can lead to better diagnostics and treatment strategies. Examples include AI-powered tools that analyze DNA sequencing data to identify rare genetic variations associated with specific diseases, ultimately leading to more precise diagnosis and targeted therapies.
Impact of Biotechnological Advancements on AI Development
Biotechnological advancements are driving the development of more sophisticated and powerful AI tools. The need to analyze biological data, particularly in genomics and proteomics, is driving the development of novel AI algorithms and techniques.
- Data Processing and Analysis: The sheer volume and complexity of biological data require sophisticated algorithms for processing and analysis. This challenge is pushing the boundaries of AI development, leading to more efficient and accurate methods for data interpretation. For instance, new machine learning models are being developed to handle the intricate details of genomic data, facilitating more effective disease prediction and diagnostics.
- Enhanced Computational Power: The demand for computational power to handle complex biological simulations and analyses is fueling the development of more powerful and efficient AI hardware. Advancements in hardware are directly responding to the increasing computational needs of bioinformatics and computational biology, allowing for faster and more precise analysis of biological systems.
Challenges of Biotech and AI Convergence
Despite the significant benefits, the convergence of biotechnology and AI presents several challenges. Addressing these issues is crucial for ensuring responsible and ethical development and application.
- Data Privacy and Security: The collection and analysis of sensitive biological and medical data raise concerns about privacy and security. Robust safeguards and regulations are essential to protect patient information. Data breaches can have devastating effects on patient privacy and trust in healthcare systems.
- Ethical Considerations: The use of AI in healthcare raises ethical considerations regarding bias in algorithms, access to technology, and the potential for misuse. Addressing these concerns proactively is vital to ensure equitable access and responsible development. Examples include ensuring that AI algorithms do not perpetuate existing biases in patient data, potentially leading to disparities in treatment.
AI in Drug Discovery and Development
Artificial intelligence (AI) is rapidly transforming the pharmaceutical industry, offering the potential to accelerate drug discovery and development, ultimately improving patient outcomes. AI algorithms can analyze vast datasets, identify patterns, and make predictions that were previously impossible for humans to achieve. This capability promises to dramatically reduce the time and cost associated with bringing new therapies to market.AI is employing sophisticated methods to sift through mountains of biological data, identify promising drug candidates, and optimize clinical trial design.
This allows researchers to focus on the most promising leads, thereby reducing the likelihood of failures in later stages of development.
Methods of AI in Drug Discovery
AI employs various methods to expedite drug discovery, including machine learning (ML) algorithms. These algorithms can identify correlations between molecular structures and biological activity, helping predict the efficacy and safety of potential drug candidates. Deep learning models, a subset of ML, can analyze complex biological data, like protein structures and genetic information, to identify patterns and insights that might otherwise be missed.
This data-driven approach has already demonstrated remarkable progress in identifying novel drug targets and accelerating lead optimization.
AI-Powered Platforms for Drug Candidate Identification
Several AI-powered platforms are emerging that leverage these methods to identify drug candidates. These platforms analyze massive datasets of molecular structures, biological activity, and clinical trial results to identify promising drug candidates. For instance, some platforms utilize algorithms to predict the binding affinity of molecules to target proteins, while others can predict the potential toxicity of a drug.
This ability to quickly analyze vast amounts of data significantly accelerates the process of identifying promising candidates. One such platform is Atomwise, which uses AI to identify small-molecule drugs that can target specific proteins associated with diseases.
Optimization of Clinical Trial Design
AI can also optimize clinical trial design, enabling more efficient and effective trials. AI algorithms can analyze patient data to identify the most suitable participants for a trial, reducing the need for extensive and expensive screening. Furthermore, AI can predict the optimal trial size and duration, minimizing risks and maximizing the efficiency of resource allocation. This predictive ability allows for more tailored and targeted trials, reducing the time and cost of clinical testing.
Ethical Considerations in AI Drug Development
The increasing reliance on AI in drug development raises several ethical concerns. One major concern is the potential for bias in AI algorithms, which can lead to the development of drugs that are not effective or safe for all patient populations. Another concern is the potential for data privacy violations if sensitive patient data is not handled responsibly.
Ensuring transparency and accountability in the use of AI algorithms is crucial to mitigating these risks. Furthermore, the potential for job displacement in the pharmaceutical industry requires careful consideration and strategic workforce development initiatives. Maintaining human oversight in the decision-making process remains vital, as AI should be seen as a tool to augment, not replace, human expertise.
AI for Personalized Medicine
AI is revolutionizing healthcare by enabling the development of personalized medicine approaches. This involves tailoring treatments to individual patient characteristics, maximizing efficacy and minimizing adverse effects. By leveraging vast datasets and sophisticated algorithms, AI can analyze patient-specific data to predict treatment responses and optimize therapeutic strategies. This approach promises to improve patient outcomes and enhance the overall efficiency of healthcare systems.
Analyzing Individual Patient Data for Tailored Treatments
AI algorithms can analyze diverse patient data, including genetic information, medical history, lifestyle factors, and environmental exposures. This comprehensive analysis allows for the identification of patterns and insights that would be challenging for human clinicians to discern. By incorporating these insights, AI can help predict individual patient responses to various treatments, enabling the selection of the most effective therapies and dosages.
This personalized approach enhances treatment efficacy and minimizes the risk of adverse reactions.
AI-Driven Diagnostic Tools for Early Disease Detection
AI-powered diagnostic tools can analyze medical images (such as X-rays, CT scans, and MRIs) to identify subtle patterns indicative of diseases. These tools can detect anomalies and potential health issues earlier than traditional methods, leading to earlier intervention and potentially better outcomes. For example, AI algorithms can analyze mammograms to detect breast cancer at earlier stages, often before symptoms manifest.
Another example is in the detection of diabetic retinopathy in retinal images, a significant cause of vision loss. These early detection capabilities can significantly improve patient prognoses.
Predicting Patient Responses to Therapies
AI can predict how patients will respond to specific therapies based on their unique characteristics and medical history. This predictive capability is particularly valuable in oncology, where patient responses to chemotherapy and targeted therapies can vary significantly. By analyzing patient data, including genetic profiles and tumor characteristics, AI can help clinicians predict which therapies are most likely to be effective for each individual patient.
This enables clinicians to select the most appropriate treatment regimen for each patient.
Developing Personalized Therapies
AI is facilitating the development of novel personalized therapies. By analyzing vast datasets of genomic information and clinical trial data, AI can identify potential drug targets and predict the efficacy of novel drug candidates. This accelerates the drug discovery process and allows for the development of therapies tailored to specific patient populations. Furthermore, AI can aid in optimizing drug delivery systems to ensure that the medicine reaches the target cells effectively.
This precision medicine approach promises to improve treatment outcomes and reduce the overall cost of healthcare.
AI in Biotech Research and Development

Artificial intelligence (AI) is rapidly transforming biotech research and development, offering unprecedented opportunities to accelerate innovation and address global challenges. AI’s ability to process and analyze vast datasets, coupled with its capacity for pattern recognition, enables researchers to unlock insights hidden within complex biological systems. This powerful synergy between AI and biotechnology is poised to revolutionize drug discovery, personalized medicine, and the development of novel biotechnologies.AI excels at sifting through the enormous quantities of biological data generated by high-throughput screening, genomics projects, and other experimental techniques.
This capability allows researchers to identify correlations and patterns that might be missed by traditional methods, potentially leading to breakthroughs in understanding biological processes and disease mechanisms.
Analyzing Large Datasets of Biological Information
AI algorithms, particularly machine learning models, are adept at extracting meaningful information from complex biological datasets. These datasets encompass genomic sequences, protein structures, clinical trial data, and more. By leveraging advanced algorithms, AI can identify correlations between genetic variations and disease susceptibility, predict drug efficacy and toxicity, and discover novel therapeutic targets.
Accelerating the Development of New Biotechnologies
AI can dramatically accelerate the development of new biotechnologies. For instance, AI-powered tools can optimize experimental designs, enabling researchers to focus their efforts on promising avenues and reduce wasted resources. This can be particularly impactful in fields like synthetic biology, where the creation of novel biological systems requires iterative design and testing. AI can also streamline the process of identifying suitable cell lines for research or manufacturing processes.
Framework for Using AI in Genomics Research
A robust framework for utilizing AI in genomics research involves several key steps. First, data collection and pre-processing are critical to ensure the quality and reliability of the input data. Second, the selection of appropriate AI algorithms, considering factors such as dataset size, complexity, and desired outcomes, is essential. Third, the interpretation and validation of AI-derived insights must be meticulously performed to ensure accuracy and avoid spurious correlations.
This iterative process of data analysis, model refinement, and validation is essential for reliable insights. A typical framework would involve (1) data cleaning and integration, (2) model training and evaluation, (3) result interpretation and validation, and (4) feedback loop for model refinement.
Comparative Analysis of AI Algorithms in Biotech Research
Different AI algorithms exhibit varying strengths and weaknesses in biotech research. A comparative analysis can help researchers select the most appropriate tool for a given task. For instance, deep learning excels at complex pattern recognition in large image datasets, making it suitable for tasks like analyzing protein structures. Support vector machines (SVMs) are effective in classification tasks, such as predicting disease risk based on genetic profiles.
A comprehensive comparison would involve evaluating factors such as accuracy, computational cost, interpretability, and robustness of each algorithm within the context of specific biological questions. A table outlining the comparative strengths and weaknesses of popular algorithms is provided below.
Algorithm | Strengths | Weaknesses |
---|---|---|
Deep Learning | Excellent pattern recognition, handles high-dimensional data | Computationally intensive, potentially less interpretable |
Support Vector Machines (SVM) | Effective for classification tasks, relatively efficient | May not perform as well with highly complex datasets |
Random Forests | Robust, handles various data types, relatively interpretable | May not capture complex relationships as effectively as deep learning |
Data Management and Analysis in Biotech and AI
Effective data management and analysis are crucial for harnessing the full potential of AI in biotech. Robust systems are needed to store, process, and interpret the massive datasets generated by various biotechnological techniques and AI algorithms. This allows for the identification of meaningful patterns and insights, ultimately accelerating research and development.Data management is no longer a peripheral concern but a foundational element in biotechnological advancements.
The convergence of AI and biotech necessitates sophisticated strategies for managing and analyzing the large, diverse, and often complex datasets generated during research.
Biotech and AI are rapidly converging, demanding powerful tools for analysis and development. To keep up with the latest breakthroughs, developers need top-tier hardware. This means investing in the best laptops for developers, like those featured in this guide Best laptops for developers , to efficiently handle complex simulations and data processing. This ultimately accelerates the pace of innovation in the biotech and AI fields.
AI-Driven Large Dataset Management in Biotech Research
AI offers powerful tools for managing and analyzing large datasets, crucial for handling the vast amounts of information in biotech. Machine learning algorithms can automate data preprocessing, filtering, and feature extraction, significantly accelerating the analysis process. This automation reduces human error and frees researchers to focus on higher-level tasks. Furthermore, AI-powered systems can identify patterns and anomalies in data that might be missed by manual analysis, leading to faster and more accurate insights.
Data Visualization for Biotech Insights
Data visualization plays a critical role in transforming complex biotech data into easily understandable insights. Visual representations such as charts, graphs, and interactive dashboards allow researchers to rapidly grasp trends, patterns, and relationships within their data. Visualizations can reveal correlations between different variables, highlighting potential therapeutic targets or biomarkers, and accelerating the identification of promising research avenues. For instance, heatmaps can visually represent gene expression levels across different conditions, facilitating the identification of key genes involved in disease progression.
Data Security and Privacy in Biotech AI Applications
Robust data security and privacy measures are essential to protect sensitive information in biotech AI applications. Data encryption, access controls, and secure storage protocols are vital components of a comprehensive security strategy. Regulations like HIPAA (Health Insurance Portability and Accountability Act) in the US, or GDPR (General Data Protection Regulation) in Europe, need to be adhered to strictly.
Furthermore, anonymization and de-identification techniques can safeguard patient privacy while still allowing for valuable research.
System Design for Organizing Biotech Data for AI Analysis
A well-structured system is essential for efficiently organizing biotech data for AI analysis. This includes a standardized data format, clear metadata, and a robust database infrastructure. A relational database structure, for example, can effectively link different datasets and facilitate complex queries. A metadata system should include detailed descriptions of data sources, collection methods, and experimental conditions. This metadata ensures data integrity and allows for effective data interpretation.
Furthermore, data should be organized into distinct, interconnected modules to reflect the specific research stages, such as data acquisition, processing, analysis, and reporting. This modular approach makes it easier to manage data and conduct analyses tailored to specific research needs.
Ethical Considerations and Societal Impact
The convergence of biotechnology and artificial intelligence presents a wealth of opportunities, yet it also raises complex ethical questions and potential societal impacts. Careful consideration of these factors is crucial to ensure responsible development and deployment of these technologies. The potential benefits, such as improved healthcare and enhanced research capabilities, must be weighed against the potential risks and societal consequences.The development and application of AI in biotechnological contexts necessitate careful evaluation of ethical implications.
Transparency, accountability, and equitable access are key considerations. The potential for bias in AI systems, and the resulting impact on diverse populations, requires proactive mitigation strategies.
Potential Biases in AI Systems
AI systems, particularly those trained on historical data, can perpetuate existing societal biases. This is particularly concerning in biotechnological applications, where decisions about drug development, diagnosis, and treatment can have profound impacts on individuals. For instance, if a dataset used to train an AI system for diagnosing a disease is skewed towards a particular demographic, the system might be less accurate or even discriminatory toward other groups.
The inherent biases present in data can lead to inaccurate predictions or treatment recommendations, thus exacerbating existing health disparities.
Importance of Transparency and Accountability
Transparency and accountability are paramount in AI-driven biotechnological applications. Understanding how AI systems arrive at their conclusions is essential for building trust and ensuring ethical use. This requires clear documentation of the algorithms, data sources, and decision-making processes. Furthermore, mechanisms for oversight and accountability are needed to address potential errors or misuse. Establishing clear lines of responsibility for the outcomes of AI-driven biotechnological interventions is critical.
Societal Impacts of Biotech and AI Convergence
The convergence of biotechnology and AI has the potential to reshape numerous aspects of society. Access to advanced medical treatments and personalized medicine could be significantly improved. However, there is also a potential for widening existing inequalities if access to these technologies is not equitably distributed. The development of new technologies for agricultural production and resource management could lead to both positive and negative impacts, depending on how they are implemented.
Ethical Implications of AI in Biotechnological Applications
The use of AI in biotechnology raises a number of ethical concerns. One crucial issue is the potential for misuse of genetic information. The ability to analyze and manipulate genetic data raises questions about privacy, consent, and the potential for discrimination. Furthermore, the development of advanced gene editing technologies raises ethical considerations regarding the alteration of human germline cells.
A thorough understanding of the societal implications is essential before implementation.
Examples of Potential Societal Impacts
Several societal impacts are possible, including:
- Increased access to healthcare: AI-powered diagnostic tools and personalized medicine approaches could potentially improve healthcare access and outcomes, especially in underserved populations.
- Ethical concerns regarding genetic information: The ability to access and analyze genetic data raises concerns about privacy, security, and the potential for misuse or discrimination based on genetic predispositions.
- Potential for widening health disparities: Unequal access to AI-driven biotechnological advancements could exacerbate existing health disparities and create new inequalities.
- Transformation of agriculture and food production: AI-powered precision agriculture could enhance agricultural efficiency and potentially lead to increased food production.
Regulatory Landscape and Policies
The convergence of biotechnology and artificial intelligence presents significant opportunities but also raises complex regulatory challenges. Establishing clear guidelines and policies is crucial to ensure responsible development and deployment of these technologies while mitigating potential risks. This necessitates a nuanced approach that balances innovation with ethical considerations and societal well-being.Existing regulations often address aspects of biotechnology and AI individually, but the interplay between these fields demands a more integrated framework.
This requires proactive policy development to anticipate and address the novel challenges arising from their combined application.
Existing Regulations
Existing regulations for biotechnology and AI, while not specifically tailored to their intersection, provide a foundation. For example, drug development regulations in many countries, like the FDA regulations in the US, already mandate rigorous testing and approval processes for new therapies. Similarly, data privacy regulations, like GDPR in Europe, aim to safeguard personal information used in AI applications.
These existing frameworks offer a starting point but are insufficient to fully address the unique aspects of the biotech-AI convergence.
Challenges in Policy Development
Developing effective policies for biotech and AI interactions presents significant challenges. The rapid pace of technological advancement often outstrips the capacity of regulatory bodies to keep up. Defining clear lines of responsibility and accountability for AI-driven biotech innovations can be complex, particularly when the decision-making process involves both human and machine components. Ensuring equitable access to these advancements is another critical consideration, requiring policies that address potential disparities in resource availability and expertise.
Furthermore, international harmonization of regulations is essential, given the global nature of these technologies.
Areas Requiring New Regulations
Several areas necessitate new regulatory frameworks to effectively manage the biotech and AI convergence. These include:
- Data governance and security: The increasing reliance on large datasets in AI-driven biotech research demands robust data governance policies to protect sensitive biological information. These policies should address issues of data access, ownership, and use while ensuring compliance with existing privacy regulations.
- AI-driven diagnostics and therapeutics: New regulations are needed to evaluate the safety and efficacy of AI-assisted diagnostics and treatments, taking into account the unique risks associated with automated decision-making in healthcare. Clear criteria for validating AI models in medical settings are essential to establish confidence in the accuracy and reliability of these tools.
- Intellectual property rights: Defining intellectual property rights for AI-generated biotech innovations is crucial to incentivize research and development while ensuring equitable sharing of benefits. This requires innovative approaches to address the complexities of ownership and attribution in collaborative research projects involving AI.
International Collaboration
International collaboration is critical for developing effective and consistent biotech and AI policies. Shared best practices and coordinated efforts to establish common standards are vital to ensure responsible innovation and address the global implications of these technologies. This collaboration should involve not only governments but also industry stakeholders, research institutions, and civil society organizations. International forums can facilitate the exchange of information and promote the development of globally applicable guidelines.
Examples of International Collaboration
The development of international guidelines for the ethical use of AI in healthcare is an example of successful international collaboration. The establishment of these guidelines demonstrates the potential for coordinated efforts to address global challenges arising from technological advancements.
Specific Applications of AI in Biotech: Biotech And AI
AI is rapidly transforming various sectors of biotechnology, offering innovative solutions to complex challenges. This advancement allows for more precise and efficient approaches in areas such as genetic engineering, protein engineering, and agricultural biotechnology. By leveraging sophisticated algorithms and vast datasets, AI systems can accelerate research and development, leading to faster breakthroughs and improved outcomes.
AI in Genetic Engineering
AI algorithms are proving invaluable in genetic engineering, particularly in the design and optimization of gene editing tools. These tools, like CRISPR-Cas9, can be employed to modify an organism’s genetic material. AI assists by predicting the potential off-target effects of gene editing, enabling researchers to select the most effective and safe sequences. Further, AI tools facilitate the analysis of large genomic datasets, leading to a deeper understanding of gene function and interactions.
The use of AI allows for a more targeted approach to gene editing, minimizing risks and maximizing efficiency.
AI in Protein Engineering
Protein engineering aims to modify proteins for specific purposes. AI plays a significant role in this field by enabling the prediction of protein structures and functionalities. This is crucial for designing proteins with improved stability, activity, or binding affinities. AI algorithms can analyze vast amounts of protein data to identify patterns and relationships, leading to the design of novel proteins with enhanced properties.
AI in Agricultural Biotechnology
AI is revolutionizing agricultural biotechnology by enhancing crop yield and resilience. AI-powered systems can analyze data from various sources, including satellite imagery, weather patterns, and soil conditions. This analysis helps predict optimal planting times, irrigation schedules, and nutrient requirements, optimizing crop yields and minimizing resource waste. Furthermore, AI can identify disease patterns in crops, allowing for timely interventions and preventing widespread outbreaks.
This proactive approach can significantly reduce losses and increase overall agricultural output.
AI in Biomanufacturing
AI is transforming biomanufacturing by streamlining processes and enhancing efficiency. By analyzing data from various stages of biomanufacturing, such as fermentation, purification, and formulation, AI algorithms can optimize conditions for maximum yield and product quality. AI-powered predictive models can forecast potential issues, enabling proactive adjustments to ensure smooth and efficient operations. These optimized processes can reduce costs and increase the overall productivity of biomanufacturing facilities.
Comparison of AI Applications in Biotech Subfields
Biotech Subfield | Genetic Engineering | Protein Engineering | Agricultural Biotechnology | Biomanufacturing |
---|---|---|---|---|
AI Application | Predicting off-target effects of gene editing, analyzing large genomic datasets | Predicting protein structures and functionalities, designing novel proteins | Analyzing data for optimal planting, irrigation, and nutrient requirements, identifying crop diseases | Optimizing fermentation, purification, and formulation, predicting potential issues |
Future Trends and Predictions
The convergence of biotechnology and artificial intelligence is poised for significant advancements in the coming years. This intersection promises revolutionary breakthroughs across numerous sectors, including healthcare, agriculture, and environmental science. Predicting the precise trajectory of this rapidly evolving field is challenging, yet several key trends and potential innovations are emerging.
Emerging Applications of AI in Biotech
AI algorithms are increasingly being employed in diverse biotechnological applications. This includes the automation of complex laboratory procedures, streamlining drug discovery processes, and enhancing the analysis of biological data. The integration of AI tools can accelerate the development of personalized therapies, tailoring treatments to individual patients based on their unique genetic profiles.
Potential Innovations in Drug Discovery and Development
The fusion of AI and biotechnology offers unprecedented potential for accelerating drug discovery and development. AI-powered platforms can analyze vast datasets of molecular structures, predict drug efficacy, and identify potential drug targets. This can lead to the development of more effective and targeted therapies, significantly reducing the time and cost associated with traditional drug discovery methods. For example, AI algorithms can analyze patient data to identify patterns and potential treatment targets for specific diseases, accelerating the development of personalized medicine approaches.
Advancements in Personalized Medicine
AI algorithms can analyze vast amounts of patient data, including genetic information, medical history, and lifestyle factors. This analysis enables the development of personalized treatment plans, optimizing therapeutic outcomes and reducing adverse effects. Furthermore, AI can enhance the prediction of individual patient responses to various treatments, allowing clinicians to tailor therapies to specific patient needs. Examples include AI-driven tools for predicting the efficacy of cancer therapies based on a patient’s tumor characteristics, leading to more effective and targeted treatments.
Impact of Emerging Technologies
Emerging technologies like CRISPR gene editing and nanotechnology are poised to significantly impact the biotech and AI convergence. These technologies, combined with AI-powered tools for data analysis and prediction, can revolutionize various fields. For example, AI algorithms can be used to optimize CRISPR gene editing protocols, leading to more precise and efficient gene therapies.
Potential Obstacles to Development, Biotech and AI
Despite the immense potential, several obstacles may hinder the advancement of biotech and AI convergence. These include data privacy concerns, regulatory hurdles, and the need for robust ethical guidelines. Ensuring the responsible development and application of these technologies is crucial to maximizing their benefits while mitigating potential risks. Furthermore, issues related to data security and the potential for misuse of AI algorithms must be carefully addressed to prevent harmful outcomes.
The need for skilled personnel to manage and interpret the output of complex AI algorithms is also a concern.
Data Management and Analysis
Managing and analyzing the vast amounts of data generated by biotechnological experiments and AI models is critical for successful convergence. Robust data management systems and advanced analytical tools are essential to derive meaningful insights and make informed decisions. The development of standardized data formats and interoperable platforms will facilitate seamless data sharing and analysis across different research institutions and companies.
Illustrative Case Studies
AI’s transformative potential in biotechnology is becoming increasingly evident through successful case studies. These showcase the efficacy of AI algorithms in tackling complex biological challenges, leading to breakthroughs in drug discovery, personalized medicine, and research efficiency. The practical applications and demonstrable improvements highlight the field’s progress and promise.
Successful Applications of AI in Drug Discovery
AI is rapidly revolutionizing drug discovery, accelerating the identification of potential drug candidates and optimizing the development process. These advancements are crucial for addressing unmet medical needs and improving patient outcomes.
Biotech and AI are rapidly converging, and the need for streamlined development is crucial. This often translates to using low-code or no-code platforms, like Low-code vs. no-code platforms , to rapidly prototype and deploy innovative solutions. This accelerates the pace of discovery and application in the field, ultimately pushing the boundaries of what’s possible in biotech research.
Case Study | AI Methodology | Impact | Methodology Overview |
---|---|---|---|
AI-powered identification of potential COVID-19 antiviral drugs | Machine learning algorithms were trained on a vast dataset of known antiviral compounds and their biological activity. The algorithms identified promising molecules that could inhibit the virus’s replication. | Accelerated the search for effective treatments and contributed to the development of potential antiviral drugs, significantly reducing the time and resources needed to discover new medications. | Data-driven approach; the model’s prediction accuracy was assessed against existing data on antiviral compounds and validated through in-vitro and in-silico studies. |
Predictive modeling for cancer drug design | Deep learning models were trained on genomic and clinical data to predict the efficacy of various cancer drugs in specific patient populations. | Improved the efficiency and accuracy of cancer drug development, leading to the identification of personalized treatment options. | AI was employed to analyze massive datasets of patient data and drug interactions. The model’s performance was validated against clinical trial data and expert opinions. |
AI-driven optimization of clinical trials | AI algorithms were utilized to analyze patient data and clinical trial designs to identify optimal trial parameters, improving recruitment rates, and reducing costs. | Streamlined the clinical trial process by optimizing the patient selection and design parameters. This approach leads to more efficient use of resources and potentially faster drug approval. | Statistical models were developed to analyze data and predict the probability of success in various clinical trial settings. This was then used to make informed decisions regarding recruitment, sample size, and endpoints. |
Impact on the Field
These case studies illustrate the significant impact AI is having on biotechnology. The accelerated drug discovery processes, more effective treatment options, and reduced costs are substantial advantages for both researchers and patients. The increased efficiency and accuracy achieved through AI contribute to a more sustainable and productive scientific environment.
Methodology Overview
The methodology used in each case study varies based on the specific application, but common themes include the use of large datasets, sophisticated machine learning algorithms, and validation through in-vitro and in-silico experiments, as well as clinical trials. The algorithms are trained to identify patterns and relationships in the data to predict outcomes or optimize processes.
Closing Notes
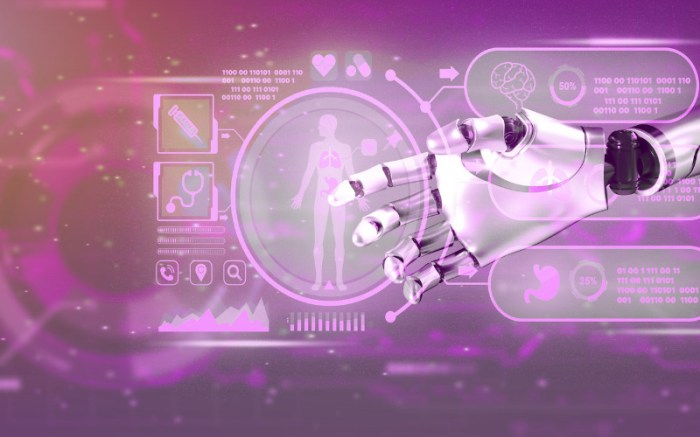
In conclusion, the fusion of biotech and AI holds immense potential for advancements in various fields, including healthcare, agriculture, and beyond. While ethical considerations and regulatory frameworks are crucial for responsible development, the collaborative spirit and innovation driving this convergence point towards a future brimming with exciting opportunities. We must carefully navigate the challenges and fully embrace the potential of this transformative technology.
FAQ Summary
What are some specific examples of AI-powered diagnostic tools?
AI is being used to develop diagnostic tools that can analyze medical images, such as X-rays and MRIs, to detect diseases like cancer at earlier stages. Machine learning algorithms are also being employed to analyze patient data to identify patterns and risk factors.
What are the primary ethical concerns regarding AI in biotech?
Ethical concerns include data privacy, potential biases in AI algorithms, and the equitable access to the benefits of this technology. Ensuring transparency and accountability in AI-powered biotech applications is paramount.
How can AI improve crop yields?
AI can analyze data from various sources, such as weather patterns, soil conditions, and plant health, to optimize crop yields. This allows for more targeted and efficient resource allocation, resulting in increased productivity.
What are the regulatory challenges in implementing biotech and AI?
Developing clear regulations for the intersection of biotech and AI is challenging due to the rapidly evolving nature of both fields. There is a need for ongoing dialogue and collaboration between stakeholders to establish appropriate guidelines and frameworks.