Brain-computer interfaces (BCIs) represent a fascinating frontier in technology, offering the potential to connect the human brain directly with computers. These interfaces are poised to revolutionize many aspects of human life, from treating neurological disorders to enhancing human-computer interaction. This exploration delves into the intricacies of BCIs, examining their technological underpinnings, applications, ethical considerations, and future prospects.
BCIs leverage various techniques to interpret and translate brain signals into commands or actions that a computer can understand. This process, while still under development, holds immense promise for individuals with disabilities, gaming, and even potentially augmenting human capabilities. The historical development of BCIs, along with the current state of research and innovation, will be thoroughly examined in the following sections.
Brain-Computer Interfaces
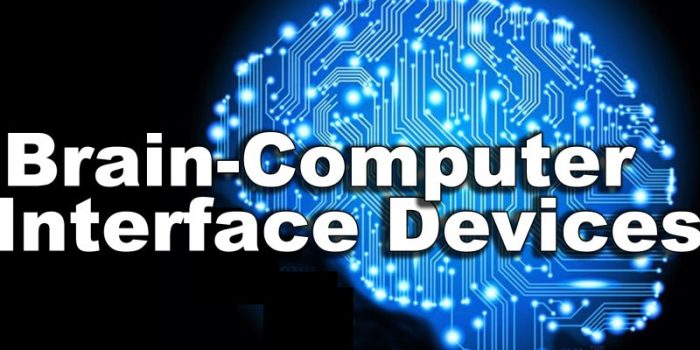
Brain-Computer Interfaces (BCIs) represent a rapidly evolving field that aims to establish direct communication pathways between the brain and external devices. This allows individuals with neuromuscular disabilities to interact with the world in new ways, and opens up possibilities for treating neurological disorders and enhancing cognitive functions.BCIs leverage the electrical signals generated by the brain, interpreting them to control external devices.
This interpretation is facilitated by sophisticated algorithms and sensors. The field is interdisciplinary, drawing upon advancements in neuroscience, computer science, and engineering.
Definition of Brain-Computer Interfaces
A Brain-Computer Interface (BCI) is a system that enables direct communication between the brain and an external device or computer. This eliminates the need for traditional input methods like muscles or nerves, enabling direct control. BCIs capture brain signals, interpret their meaning, and translate them into commands for external actions.
Fundamental Principles of BCI Technology
BCI technology rests on the fundamental principles of neural activity. Brain activity generates electrical signals, which can be measured and interpreted. Electroencephalography (EEG) and other neuroimaging techniques play a crucial role in capturing these signals. Signal processing algorithms then analyze the signals to extract relevant information, like intention or motor commands.
Types of Brain-Computer Interfaces and Their Applications
BCIs are broadly categorized by the type of brain signal they measure and the methods they employ for interpreting these signals.
- EEG-based BCIs: These BCIs rely on EEG to detect brainwaves, and are commonly used for applications like controlling cursors or prosthetic limbs. Their non-invasive nature makes them suitable for diverse applications, but their sensitivity to environmental noise and the limited spatial resolution of EEG signals can pose challenges.
- Electrocorticography (ECoG)-based BCIs: ECoG involves placing electrodes directly onto the surface of the brain. This provides higher spatial resolution than EEG, allowing for more complex control. This technique is often employed in clinical settings for specific neurological conditions, such as epilepsy, and in research for precise brain-computer interaction.
- Functional Magnetic Resonance Imaging (fMRI)-based BCIs: fMRI measures changes in blood flow in the brain. While not as fast as EEG or ECoG, fMRI provides high spatial resolution, useful for understanding cognitive processes, and has been used in research for decoding thoughts and intentions.
- BCIs using invasive methods: Invasive BCIs involve implanting electrodes directly into the brain. This allows for precise and high-speed signal capture, and is being explored for applications like restoring lost motor function. These methods have greater precision but involve greater risk and complexity.
Historical Development of BCIs
The development of BCIs has been gradual, marked by advancements in neurotechnology. Early research focused on identifying brain signals related to movement and intention. The 1970s saw early experiments using EEG to control robotic arms. The 1990s witnessed significant progress in signal processing algorithms and neuroimaging techniques.
Current State of BCI Research and Innovation
Current BCI research focuses on improving accuracy, speed, and user-friendliness. Researchers are exploring new signal processing techniques to reduce noise and enhance the decoding of complex intentions. There is also a growing interest in closed-loop BCIs, which allow for real-time interaction and feedback between the brain and the external environment. Applications are expanding beyond simple control tasks, with studies exploring cognitive enhancement and communication in individuals with severe disabilities.
Further advancements in BCIs will likely contribute to better treatment and quality of life for people with neurological conditions.
BCI Technology Overview
Brain-computer interfaces (BCIs) represent a rapidly evolving field with the potential to revolutionize human-computer interaction. They allow individuals to communicate and control external devices directly through their brain activity, bypassing traditional input methods. This technology has the potential to empower individuals with disabilities and enhance human capabilities in diverse applications.Signal acquisition is a crucial initial step in BCI technology.
Different methods measure brain activity with varying degrees of precision and cost. Understanding these techniques is fundamental to evaluating their suitability for specific applications.
Signal Acquisition Methods
Various methods are employed to capture brain signals for BCIs. Electroencephalography (EEG) measures electrical activity at the scalp, while magnetoencephalography (MEG) detects magnetic fields generated by neuronal activity. Functional near-infrared spectroscopy (fNIRS) uses near-infrared light to measure changes in blood oxygenation levels in the brain.
- EEG (Electroencephalography): EEG is a widely used non-invasive technique that records electrical activity from the scalp. It offers a relatively low cost and portability, making it suitable for various applications. However, EEG signals are susceptible to noise from external sources, resulting in lower spatial resolution compared to other methods.
- MEG (Magnetoencephalography): MEG measures magnetic fields generated by brain activity. It provides excellent temporal resolution and higher spatial resolution than EEG, allowing for better localization of brain activity. However, MEG systems are significantly more expensive and less portable than EEG systems.
- fNIRS (Functional Near-Infrared Spectroscopy): fNIRS uses near-infrared light to measure changes in blood oxygenation levels in the brain. It offers a good balance between spatial and temporal resolution, while being less susceptible to noise from external sources compared to EEG. However, fNIRS has limited depth penetration and is susceptible to scattering of light.
Signal Processing Techniques
Extracting meaningful information from brain signals requires sophisticated signal processing techniques. These techniques transform raw data into interpretable representations, facilitating communication with external devices.
- Signal Filtering: Noise reduction is a critical step in signal processing. Filtering techniques remove unwanted frequencies and artifacts from the raw EEG data. For example, specific frequencies related to muscle activity or eye movements can be filtered out to improve the signal quality.
- Feature Extraction: The extracted features represent relevant information from the brain signals, such as specific patterns of activity related to specific intentions or thoughts. Common feature extraction methods include time-domain features (e.g., average power, variance), frequency-domain features (e.g., power spectral density), and wavelet transforms.
- Classification: Machine learning algorithms are used to classify brain signals into distinct categories. This step maps brain signals to specific commands or intentions, enabling communication with external devices.
Comparison of BCI Systems
A table summarizing key characteristics of different BCI systems provides a clear overview of their strengths and weaknesses. This comparison considers accuracy, cost, and ease of use.
BCI System | Accuracy | Cost | Ease of Use |
---|---|---|---|
EEG-based BCI | Moderate to high, depending on the specific application | Low to moderate | Moderate |
MEG-based BCI | High | High | Moderate |
fNIRS-based BCI | Moderate | Moderate | Moderate |
Applications of BCIs
BCIs are poised to transform various fields by enabling direct communication and control. The diverse range of applications is highlighted in the table below.
Field | Application |
---|---|
Healthcare | Prosthetic control, communication for individuals with paralysis, monitoring neurological conditions |
Gaming | Controlling video games and virtual reality environments with thoughts |
Accessibility | Enabling communication and control for individuals with disabilities |
Research | Investigating brain function, mapping cognitive processes |
BCI Applications and Use Cases
Brain-computer interfaces (BCIs) are rapidly evolving, promising transformative applications across diverse fields. Their ability to bridge the gap between the brain and external devices holds immense potential for enhancing human capabilities and addressing various needs. From restoring lost functions to revolutionizing human-computer interaction, BCIs are poised to significantly impact the future.BCIs are not merely theoretical concepts; ongoing research and development are actively shaping their practical implementation.
Initial applications are already demonstrating the potential for improving quality of life for individuals with disabilities and expanding the horizons of human-computer interaction.
Brain-computer interfaces are rapidly evolving, pushing the boundaries of human-machine interaction. These advancements are driving a need for innovative interfaces, potentially influencing future web development trends. For instance, consider how Web development trends are adapting to accommodate the unique demands of users with disabilities, or how interactive elements might be incorporated to better reflect the capabilities of such interfaces.
This integration of cutting-edge technology will likely continue to reshape the field of brain-computer interfaces.
Healthcare Applications
BCIs are showing significant promise in healthcare, particularly in neuroprosthetics and rehabilitation. Neuroprosthetics aim to restore lost sensory and motor functions by directly connecting the brain to prosthetic limbs or devices. This allows individuals with paralysis to control robotic arms or other assistive technology through their thoughts. Rehabilitation applications leverage BCIs to assist patients in regaining motor skills after strokes or other neurological injuries.
These interfaces provide targeted feedback and training to aid in physical therapy and improve functional outcomes.
Gaming and Entertainment Applications
BCIs are finding novel applications in gaming and entertainment. The ability to translate brain activity into game controls offers exciting possibilities for immersive experiences. Imagine controlling a virtual environment or interacting with characters by simply thinking. Such applications may lead to a more intuitive and engaging form of human-computer interaction, revolutionizing gaming and entertainment.
Human-Computer Interaction Applications
BCIs are poised to reshape human-computer interaction, enabling more natural and intuitive ways to interact with technology. Imagine controlling devices with your thoughts, browsing the web using brain signals, or interacting with software applications without the need for traditional input methods. This could have profound implications for accessibility and usability.
Improving Human Capabilities, Brain-computer interfaces
BCIs are not just about restoring or enhancing existing functions; they also have the potential to significantly improve human capabilities. For instance, enhanced cognitive functions through brain-training applications or improved learning efficiency through direct neural feedback are areas where BCIs are expected to make a substantial impact.
BCI Tailoring for Specific Tasks
| BCI Type | Primary Task | Description | Example ||—|—|—|—|| Electroencephalography (EEG) based BCI | Motor imagery, mental tasks | Measures brainwaves to detect patterns associated with specific tasks. | Controlling a cursor on a screen using thought || Electrocorticography (ECoG) based BCI | Complex tasks, high precision | Measures electrical activity directly from the brain’s surface. | Controlling a prosthetic limb with high precision and speed.
|| Functional Magnetic Resonance Imaging (fMRI) based BCI | High-level cognitive tasks | Measures blood flow changes in the brain to detect specific neural activity. | Decoding complex thoughts or emotions. || Invasive BCIs | High-level, complex tasks requiring direct brain-machine interfaces | Directly connect the brain to external devices using implanted electrodes. | Controlling robotic limbs or devices with high accuracy |
Ethical Considerations of BCIs
Brain-computer interfaces (BCIs) hold immense promise for treating neurological disorders and enhancing human capabilities. However, their development and deployment raise significant ethical concerns that must be carefully addressed to ensure responsible and equitable use. These considerations span from the potential for human augmentation to societal impacts and legal frameworks.The ethical landscape surrounding BCIs is complex, encompassing issues of autonomy, privacy, and potential for misuse.
The ability to directly interface with the brain introduces new dimensions to existing ethical debates about human enhancement, impacting not only individual users but also society as a whole.
Human Augmentation
The potential for BCIs to augment human capabilities raises ethical concerns regarding the fairness and equity of access. Unequal access to this technology could exacerbate existing societal inequalities, creating a digital divide where only those with resources can benefit. This possibility underscores the importance of equitable access and responsible development strategies. A careful consideration of the long-term consequences of human augmentation is paramount.
Privacy Concerns
BCIs provide access to a vast amount of neural data, potentially revealing sensitive personal information about thoughts, emotions, and intentions. Robust data protection measures and ethical guidelines are essential to safeguard user privacy. Careful attention to the security of BCIs and the storage and handling of neural data are crucial for ensuring confidentiality. Strict regulations on data access and use are necessary to prevent unauthorized access or misuse.
Societal Impacts
Widespread BCI adoption could lead to profound societal changes, impacting employment, social interaction, and even political discourse. The potential for BCIs to influence human behavior and decision-making warrants careful examination. The societal impact of widespread BCI adoption is a complex and multi-faceted issue requiring careful consideration. BCI-related job displacement and changes in social interaction patterns are examples of potential societal impacts that should be studied and addressed proactively.
Legal Frameworks
The development of appropriate legal frameworks is crucial for regulating the use of BCIs. Laws governing data privacy, intellectual property, and the safety of medical devices need to be adapted to address the unique challenges posed by BCIs. The legal and regulatory landscape for BCIs is still evolving, and clear guidelines are necessary to address liability and accountability issues.
The lack of comprehensive legal frameworks for BCIs presents challenges in ensuring responsible use.
Potential Biases and Discrimination
BCIs could potentially perpetuate or amplify existing societal biases, leading to discrimination based on factors such as race, gender, or socioeconomic status. The design and implementation of BCIs must incorporate mechanisms to mitigate these risks. Preemptive measures to address potential biases in BCI development are critical to ensure fair and equitable use. Ensuring equitable access to BCI technology and mitigating potential biases in algorithms used for data analysis and interpretation are essential steps.
Challenges and Future Directions of BCIs
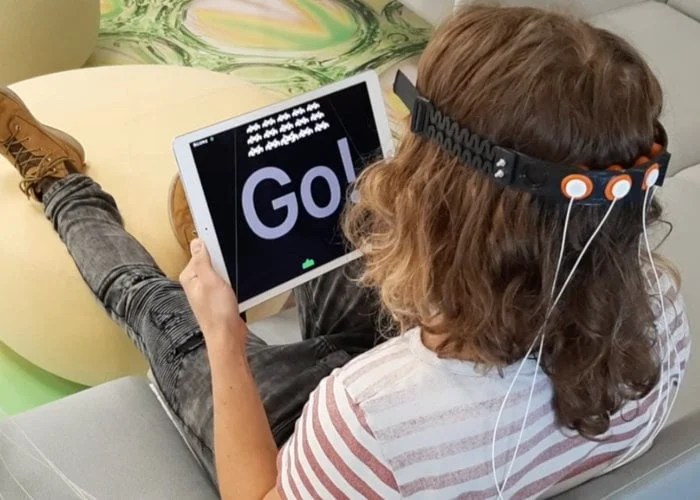
Brain-computer interfaces (BCIs) are poised to revolutionize human interaction with technology, but significant challenges remain in their development and widespread adoption. Overcoming these obstacles requires a multi-faceted approach, encompassing technological advancements, ethical considerations, and public acceptance. This section will delve into the limitations, ongoing research, and potential future of BCI technology.Current limitations in BCI technology hinder their broader application.
The limited capabilities of current BCIs often restrict the types of commands or tasks they can perform, which directly impacts the user experience. Furthermore, long-term stability and reliability are significant concerns.
Current Limitations in BCI Technology
Several key limitations currently hinder the widespread adoption of BCIs. These limitations encompass both the technical aspects and the broader societal implications. The limited signal quality and variability of neural signals make precise decoding challenging. The required invasive procedures, often involving surgical implantation, restrict accessibility and introduce potential risks. Furthermore, the lack of standardized protocols and data formats across different BCI systems hinders interoperability and research reproducibility.
Ongoing Research and Development Efforts
Researchers are actively pursuing strategies to overcome these limitations. Novel signal processing techniques are being developed to enhance the accuracy and reliability of neural signal decoding. Non-invasive BCIs are gaining traction, reducing the need for invasive procedures and expanding access. Improved electrode designs and materials are being explored to enhance signal quality and stability. Development of more user-friendly interfaces is crucial to improve the ease of use and accessibility for broader populations.
Further research is dedicated to establishing standardized protocols and data formats to promote interoperability and reproducibility in BCI research.
Potential Future Advancements in BCI Technology
The future of BCIs holds exciting possibilities. Increased computational power and advanced machine learning algorithms are expected to enhance the precision and sophistication of neural signal decoding. The development of more sophisticated and user-friendly interfaces is expected to allow users to control more complex tasks and devices. Non-invasive BCIs are expected to become increasingly prevalent, paving the way for broader accessibility.
The integration of BCIs with artificial intelligence (AI) could unlock even more transformative possibilities, enabling users to interact with technology in ways previously unimaginable.
Emerging Trends in the Field of BCIs
Several trends are shaping the future of BCI technology. The development of closed-loop BCIs, where the system dynamically adjusts its responses based on real-time user feedback, is a significant trend. This feedback loop allows for more natural and intuitive control. Another emerging trend involves the integration of BCIs with virtual reality (VR) and augmented reality (AR) environments, which opens up new possibilities for immersive experiences and therapeutic applications.
The growing interest in BCIs for rehabilitation and assistive technologies is another prominent trend, with potential applications in restoring lost motor functions.
Potential Solutions to Address the Challenges Faced by BCIs
To address the limitations and unlock the full potential of BCIs, several strategies are being implemented. The development of robust signal processing techniques, specifically those tailored for decoding complex neural signals, will improve the accuracy and reliability of BCIs. The continued refinement of non-invasive BCI technologies will broaden access and minimize the associated risks. Improved user interfaces will enhance the ease of use and improve user acceptance.
Furthermore, collaborative research efforts and standardized protocols are crucial for accelerating progress and ensuring reproducibility in the field. Addressing ethical considerations, including data privacy and potential misuse, is essential for ensuring the responsible development and deployment of BCIs.
Signal Acquisition and Processing Techniques
Brain-computer interfaces (BCIs) rely heavily on accurate and efficient signal acquisition and processing to translate neural activity into actionable commands. This process involves capturing electrical, magnetic, or optical signals from the brain and then extracting meaningful information from the raw data. Different signal acquisition methods have distinct advantages and limitations, and signal processing plays a crucial role in filtering noise and extracting relevant information from the acquired signals.
Physiological Principles of EEG, MEG, and fNIRS
Electroencephalography (EEG), magnetoencephalography (MEG), and functional near-infrared spectroscopy (fNIRS) are the primary techniques used to acquire brain signals for BCIs. Understanding the underlying physiological principles behind these techniques is vital for interpreting the acquired data. EEG measures the electrical activity of populations of neurons, MEG detects the magnetic fields produced by these electrical currents, and fNIRS assesses changes in blood oxygenation levels related to neural activity.
EEG Signal Acquisition and Processing
EEG signal acquisition involves placing electrodes on the scalp. The electrodes measure the voltage fluctuations generated by neuronal activity. The signal is amplified and filtered to remove noise from environmental sources. Signal processing steps such as filtering, artifact rejection, and independent component analysis (ICA) are crucial to extract the relevant brain activity.
MEG Signal Acquisition and Processing
MEG measures the magnetic fields produced by electrical activity in the brain. The measurement requires a highly sensitive magnetometer and a shielded room to minimize external magnetic interference. The signal is typically processed using techniques similar to EEG, such as filtering and artifact removal, but with specific considerations for the magnetic nature of the signal.
fNIRS Signal Acquisition and Processing
fNIRS uses near-infrared light to measure changes in blood oxygenation levels in the brain. Optical sensors are placed on the scalp, and changes in light absorption reflect variations in blood flow, indirectly correlating with neural activity. Signal processing for fNIRS involves analyzing the detected light intensity changes to identify regions of increased or decreased blood oxygenation.
Signal Processing Pipeline in a Typical BCI System
A typical signal processing pipeline for a BCI system follows a structured approach. The pipeline starts with signal acquisition, followed by pre-processing steps to remove artifacts and noise. Then, feature extraction identifies patterns and characteristics within the data. Finally, classification algorithms convert the extracted features into actionable commands. The following flowchart illustrates this pipeline:
+-----------------+ +-----------------+ +-----------------+ | Signal Acquisition |----->| Pre-processing |----->| Feature Extraction| +-----------------+ +-----------------+ +-----------------+ | EEG, MEG, | | Filtering, | | Pattern | | fNIRS | | Artifact Rejection| | Recognition | +-----------------+ +-----------------+ +-----------------+ | | ICA, | | | | | Baseline | | | | | Correction | | | | +-----------------+ | | V V | +-----------------+ +-----------------+ | Classification | | Action Output | +-----------------+ +-----------------+ | V BCI Output
Real-World BCI Application Example
A notable application using EEG-based BCI is controlling a robotic arm.
By identifying distinct patterns in EEG signals associated with specific movements, the system can translate user intentions into precise robotic arm motions. Signal processing techniques like Independent Component Analysis (ICA) are crucial in this context for isolating brain activity related to motor commands from background noise.
Hardware Components of BCIs
Brain-computer interfaces (BCIs) rely on a sophisticated interplay of hardware components to translate neural signals into commands or actions. These components must be carefully chosen and integrated to ensure reliable signal acquisition, processing, and transmission. Understanding the intricacies of these components is crucial for designing effective and user-friendly BCI systems.
The diverse hardware components in a BCI system work in concert to acquire, process, and interpret neural signals. From the electrodes that directly interact with the brain to the sophisticated signal processing units, each element plays a vital role in translating thoughts into actions. The overall system architecture is crucial for both functionality and user experience.
Electrode Types for Signal Acquisition
Different types of electrodes are used for acquiring neural signals, each with its own strengths and weaknesses. The choice of electrode type depends on the specific application and the depth of brain tissue that needs to be monitored.
- Electrocorticography (ECoG) electrodes are placed directly on the surface of the brain, typically during neurosurgical procedures. These electrodes provide high-resolution signals, but their use is limited to patients undergoing surgery and require careful surgical placement. Their high resolution makes them suitable for tasks requiring fine motor control.
- Microelectrodes, such as those used in microelectrode arrays (MEAs), are significantly smaller than ECoG electrodes and can be implanted into the brain tissue to record from specific neuronal populations. Their smaller size allows for recording from a more precise area, providing detailed insights into neural activity, but require precise placement and can have limited access.
- Electroencephalography (EEG) electrodes are placed on the scalp, recording electrical activity from the brain’s surface. EEG is a non-invasive technique, making it suitable for a wider range of users. However, the signals are less precise and can be more susceptible to noise, which limits the precision of the output.
Signal Processing Hardware
Signal processing hardware is critical in translating raw neural signals into meaningful information. Different types of hardware offer varying levels of processing capabilities and speed.
- Analog signal processing circuits are essential for initial signal conditioning and amplification. These circuits convert the weak electrical signals from the electrodes into a format suitable for further processing. They are cost-effective but have limitations in terms of signal processing complexity.
- Digital signal processing (DSP) units are used for more complex signal processing tasks, such as filtering, feature extraction, and classification. These units are highly flexible and allow for sophisticated algorithms to be implemented for improved signal interpretation and provide greater control over the output, but their high complexity might lead to more intricate hardware.
BCI System Architecture
The architecture of a BCI system encompasses all the hardware and software components involved. A well-designed architecture ensures seamless communication and efficient processing of neural signals.
(A placeholder for a diagram. The diagram would show a simplified schematic of a BCI system, including the signal acquisition device (e.g., electrodes), signal processing unit (e.g., amplifier, DSP), communication interface (e.g., wireless transmitter), and the user interface (e.g., computer screen or robotic arm) connected to the signal processing unit. The components would be linked by arrows to illustrate the flow of signals. The diagram would clearly show the input and output pathways of the system.)
- Signal Acquisition: Electrodes detect the neural activity, which is then amplified and conditioned.
- Signal Processing: The conditioned signal undergoes filtering, feature extraction, and classification to isolate relevant information.
- Communication Interface: This module enables communication between the signal processing unit and the user interface.
- User Interface: This component translates the processed signals into meaningful actions, such as controlling a cursor or a robotic arm.
Software Components of BCIs: Brain-computer Interfaces
Brain-computer interfaces (BCIs) rely heavily on sophisticated software to process the neural signals captured by hardware components and translate them into meaningful actions or outputs. This software acts as the bridge between the biological brain and the external world, enabling communication and control beyond traditional methods. The complexity of this software is crucial for the effective operation and reliability of a BCI system.
The software components of a BCI system are designed to perform a series of tasks, from signal preprocessing to decoding and output generation. The efficiency and accuracy of these components directly impact the performance and utility of the overall BCI system. This intricate interplay between hardware and software is essential for realizing the full potential of BCI technology.
Brain-computer interfaces (BCIs) are rapidly evolving, and their potential is huge. Machine learning applications are playing a crucial role in advancing BCI technology, offering sophisticated algorithms for decoding neural signals. These advancements are driving innovation in BCIs, enabling more precise control and interaction with the outside world. Machine learning applications are transforming the way we approach BCIs, promising greater functionality and usability for patients with disabilities.
Different Software Components
The software in a BCI system is composed of several distinct modules, each with its own specific function. These modules work in concert to process the neural signals, interpret the user’s intent, and generate the desired output.
- Signal Preprocessing: This module prepares the raw neural signals for further analysis. It involves steps like filtering, noise reduction, and artifact removal. This crucial initial step ensures the quality of the data fed into subsequent analysis stages. Without proper preprocessing, the accuracy of the BCI system will be compromised.
- Feature Extraction: This module identifies meaningful patterns and characteristics (features) within the preprocessed neural signals. These features, which can be represented as numerical values or other data structures, encapsulate essential information about the user’s intent or brain activity. Examples of features include signal amplitude, frequency, and latency. Extracted features are critical for accurate classification and decoding.
- Classification/Decoding: This module interprets the extracted features to classify the user’s intent or predict the desired output. Machine learning algorithms are commonly employed in this stage to learn patterns in the data and map them to specific actions or commands. This step allows the BCI system to understand what the user wants to do.
- Output Generation: This module takes the decoded information and translates it into a usable output. This might involve controlling a robotic arm, typing on a keyboard, or stimulating a prosthetic limb. The specific output method depends on the application of the BCI.
Role of Machine Learning Algorithms
Machine learning (ML) algorithms play a vital role in BCI systems, particularly in the classification and decoding stages. These algorithms learn from the input data and develop a model that can accurately predict the user’s intent based on their brain activity.
- Model Training: The training process involves feeding the ML algorithm with labeled data. This data consists of neural signal recordings paired with corresponding user actions or intents. The algorithm learns to associate specific patterns in the signals with particular outputs. This process is iterative and requires careful selection and preparation of the training dataset.
- Model Evaluation: The trained model is evaluated to assess its performance. This involves testing the model with new, unseen data and measuring its accuracy, precision, and other relevant metrics. A well-performing model is crucial for reliable BCI operation.
- Model Selection: Various machine learning algorithms are available for BCI applications. The choice of algorithm depends on factors such as the type of neural signals being analyzed, the desired output, and the complexity of the task. Commonly used algorithms include support vector machines (SVMs), artificial neural networks (ANNs), and decision trees.
Data Analysis in BCI Systems
Data analysis is crucial for optimizing BCI systems and ensuring their reliability. The collected data is analyzed to identify patterns, trends, and outliers that can inform the design and operation of the BCI.
- Data Visualization: Data visualization techniques are employed to present complex data in an easily understandable format. This allows researchers and clinicians to quickly identify patterns and anomalies in the data, which can provide insights into the user’s brain activity.
- Performance Metrics: Performance metrics are used to quantify the success of a BCI system. These metrics include accuracy, precision, and recall. These metrics help evaluate the efficiency of the BCI in translating brain signals into actions. The goal is to improve these metrics to enhance the usability of the BCI.
- Statistical Analysis: Statistical methods are applied to analyze the data and identify significant correlations between neural signals and user actions. This statistical analysis helps determine the reliability and significance of the results obtained from the BCI.
User Interaction and Feedback Mechanisms
Brain-computer interfaces (BCIs) require intuitive and effective user interaction to be truly impactful. This section delves into the methods users employ to command BCIs, the feedback mechanisms that inform their actions, and the crucial role of training in optimizing BCI performance. Understanding these aspects is essential for advancing BCI technology and realizing its potential across diverse applications.
User Interaction Methods
BCI systems rely on various methods for users to communicate their intentions. These methods can be broadly categorized as direct and indirect. Direct methods involve mapping brain signals directly to desired actions, while indirect methods utilize intermediary steps. The choice of method often depends on the specific application and the nature of the desired output.
- P300 Speller: This method capitalizes on the P300 brainwave response, a specific electrical signal associated with cognitive processing. By presenting a matrix of characters, users mentally select a character, triggering a P300 response, which the system translates into a character selection.
- Motor Imagery: Users visualize performing a specific movement without actually executing it. The system interprets these mental rehearsals to generate corresponding commands. This method finds application in controlling prosthetic limbs and robotic devices.
- Event-Related Potentials (ERPs): ERPs are electrical brain signals associated with specific cognitive events. By detecting these signals, BCIs can interpret user intentions. Different types of ERPs, like those related to attention or decision-making, can be used to elicit specific responses.
- Electrocorticography (ECoG): ECoG measures brain activity directly from the surface of the exposed brain. This method provides a high-resolution signal and can be used for more complex tasks, but it carries higher risks due to the invasive nature.
Feedback Mechanisms
Effective feedback is crucial for user training and performance optimization. BCI systems must provide users with clear indications of their commands and the system’s response.
- Visual Feedback: Displaying visual cues, such as changing colors or moving cursors, on a screen allows users to monitor the system’s interpretation of their brain activity. This visual representation directly correlates the brain activity to the visual feedback.
- Auditory Feedback: Auditory cues, such as beeps or tones, provide aural feedback to inform users about their commands. This is particularly helpful in applications where visual feedback is not possible or sufficient.
- Haptic Feedback: Physical feedback, like vibrations or movements, provides a tactile response to inform users about their commands, useful for applications requiring motor control, such as controlling robotic arms.
User Training for BCIs
Proper training is essential for users to effectively interact with BCI systems. Training programs vary based on the specific BCI technology and the intended application.
- Practice and Repetition: Consistent practice and repetition are fundamental to improving user proficiency. Repetitive tasks and simulations allow users to refine their mental commands.
- Personalized Feedback: Adjusting feedback to individual user responses is vital for optimal performance. Tailoring the training process based on individual strengths and weaknesses leads to faster learning curves.
- Progressive Complexity: Introducing tasks of increasing complexity progressively builds user skill and confidence in using the BCI. Starting with simpler tasks and gradually moving towards more complex ones is crucial.
Examples of BCI User Interfaces
Various user interfaces have been developed to facilitate BCI interaction. These interfaces range from simple text input systems to more complex control interfaces.
- Text Input: BCIs can be used to type text by mapping brain activity to specific character selections. This is often used for communication tasks.
- Cursor Control: Users can control a cursor on a screen using their brain activity, enabling them to interact with computer applications and perform tasks like navigation.
- Prosthetic Control: BCIs can be used to control prosthetic limbs, allowing individuals to interact with their environment and perform tasks like grasping objects.
Comparison of User Interaction Methods
Method | Description | Advantages | Disadvantages |
---|---|---|---|
P300 Speller | Selects characters using P300 brainwaves. | Relatively simple to learn, accurate. | Can be slow, requires focused attention. |
Motor Imagery | Visualizes movements to control devices. | Intuitive, potentially high accuracy. | Requires practice, mental focus, susceptible to artifacts. |
ERPs | Uses ERPs to interpret user intentions. | Can be fast, adaptable to different tasks. | Requires specific ERP types, can be complex. |
ECoG | Measures brain activity directly from the exposed brain. | High resolution, potentially complex tasks. | High risk, invasive, requires specialized equipment. |
Clinical Applications of BCIs
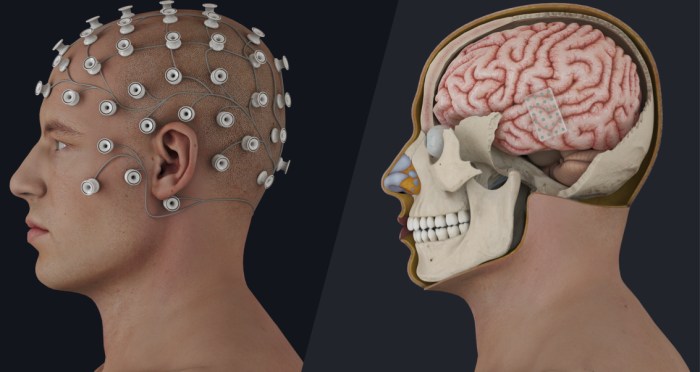
Brain-computer interfaces (BCIs) hold immense promise for revolutionizing the treatment of neurological disorders. Their ability to bypass damaged neural pathways and directly communicate with the brain opens up new avenues for restoring lost functions and improving the quality of life for individuals affected by debilitating conditions. This section explores the potential of BCIs in clinical settings, focusing on specific applications and the key factors influencing successful implementation.
Potential in Treating Neurological Disorders
BCIs offer a transformative approach to treating a wide range of neurological disorders. By enabling direct communication between the brain and external devices, BCIs can restore lost motor functions, alleviate symptoms, and improve overall cognitive performance. The development of more sophisticated and user-friendly BCI systems is crucial for their widespread clinical application.
BCI Applications in Stroke Rehabilitation
Stroke is a leading cause of neurological disability, often resulting in motor impairments and paralysis. BCIs show considerable promise in stroke rehabilitation. By detecting and interpreting brain signals associated with intended movements, BCIs can allow individuals to control robotic arms or other assistive devices, facilitating the recovery of lost motor skills.
BCI Applications in Paralysis
For individuals with paralysis, BCIs offer a pathway to regain independence and control over their environment. BCI systems can be trained to recognize the brain signals associated with desired actions, allowing users to operate computer cursors, prosthetic limbs, or even communicate through text-to-speech systems. Successful cases have demonstrated the potential of BCIs to enhance the quality of life for individuals with paralysis.
Restoring Lost Motor Functions
BCIs can restore lost motor functions by bridging the gap between the brain and the muscles. By detecting and decoding neural signals related to intended movements, BCIs allow users to control external devices, such as robotic limbs or computer cursors. This capability offers a significant opportunity to rehabilitate patients with neurological impairments and enhance their functional independence.
Potential in Treating Other Neurological Conditions
Beyond stroke and paralysis, BCIs demonstrate potential in treating other neurological conditions, including:
- Amyotrophic Lateral Sclerosis (ALS): BCIs can provide communication channels for individuals with ALS, enabling them to interact with the world despite significant muscle deterioration.
- Parkinson’s Disease: BCIs could potentially alleviate motor symptoms by providing real-time adjustments to tremor and rigidity.
- Epilepsy: BCIs may offer the capability to predict and potentially prevent seizures by detecting pre-ictal brain activity.
- Spinal Cord Injury: BCIs could help restore lost motor function and sensory feedback in individuals with spinal cord injuries.
Critical Factors for Successful Implementation
Successful BCI implementation in clinical settings requires careful consideration of several key factors:
- Safety and Reliability: BCIs must be designed with safety and reliability in mind to prevent harm and ensure consistent performance.
- User Experience: BCI systems should be user-friendly and intuitive to ensure effective and comfortable use by patients.
- Long-Term Compatibility: BCIs should be designed to be compatible with the patient’s changing needs over time, and should be adaptable to long-term use.
- Ethical Considerations: BCIs raise important ethical concerns regarding privacy, autonomy, and access to technology. These issues need to be addressed proactively during development and implementation.
Examples of Successful Applications
Several ongoing clinical trials and real-world case studies demonstrate the effectiveness of BCIs in treating neurological disorders. These studies illustrate the potential for BCIs to improve quality of life and restore lost functions for individuals with neurological impairments.
Illustrative Examples of BCI Applications
Brain-computer interfaces (BCIs) are rapidly evolving, showcasing diverse applications beyond initial clinical trials. These systems promise to revolutionize human-computer interaction, offering new avenues for communication and control for individuals with disabilities, and potentially expanding into other areas. From controlling prosthetic limbs to gaming and entertainment, BCIs are demonstrating their potential to reshape daily life.
Prosthetic Limb Control
BCIs are being investigated extensively for use in controlling prosthetic limbs. This application leverages neural signals to provide precise and intuitive control over the movement of prosthetic devices. Users can translate their thoughts and intentions into actions, potentially restoring a sense of natural movement and independence.
- Mechanism: Electroencephalography (EEG) or electromyography (EMG) signals are collected from the user’s muscles or brain. Advanced algorithms analyze these signals to identify the user’s intent to move a specific part of the prosthetic limb. The identified signals are then used to activate the corresponding motor movements in the prosthetic limb.
- Benefits: This allows users to regain a degree of control over their prosthetic limbs, potentially improving their quality of life by enabling more natural and intuitive interactions with the environment. The improved precision and control over the prosthetic limb enhance the user’s ability to perform daily tasks.
- Example: Researchers have developed BCI systems that allow individuals with paralysis to control prosthetic hands with remarkable accuracy. These systems enable them to perform tasks like grasping objects, opening doors, and writing.
Communication for Individuals with Disabilities
BCIs can offer alternative communication pathways for individuals with severe motor impairments. These systems can directly translate brain activity into text or speech, enabling them to interact with the world and communicate with others.
- Mechanism: BCI systems designed for communication typically employ EEG to detect patterns in brain activity associated with specific words or phrases. The identified patterns are translated into text or speech output.
- Benefits: This provides a new avenue for communication, enhancing independence and enabling individuals with severe disabilities to participate more fully in society.
- Example: Individuals with locked-in syndrome have demonstrated the ability to communicate with others using BCIs. These systems allow them to express their thoughts and feelings, bridging the communication gap that their physical limitations create.
Gaming and Entertainment
The entertainment industry is exploring the potential of BCIs for creating innovative gaming experiences. Users can control game elements and characters directly with their thoughts, adding an entirely new dimension to interactive entertainment.
- Mechanism: BCI systems in gaming environments often rely on EEG or other neural signals to detect and translate user intentions into game actions. The user’s thoughts about desired actions are translated into game commands.
- Benefits: This can lead to more immersive and intuitive gaming experiences. It can be especially beneficial for individuals with limited mobility or those who want a more natural and intuitive way to interact with virtual worlds.
- Example: Several companies are developing BCI-enabled gaming systems. Players can control game elements, like character movement or weapon selection, simply by thinking about the desired action.
Table of Real-World BCI Use Cases
Application Area | Description | Key Features |
---|---|---|
Prosthetic Control | Controlling prosthetic limbs using brain signals. | Precise movement, intuitive control. |
Communication | Alternative communication for individuals with disabilities. | Direct brain-to-text/speech translation. |
Gaming | Controlling game elements with brain activity. | Intuitive and immersive gaming experience. |
Case Study: BCI-Controlled Wheelchair
A significant application of BCI technology is the development of brain-controlled wheelchairs for individuals with paralysis. These wheelchairs enable users to navigate their surroundings and maintain mobility by directly translating their brain activity into commands. The system tracks the user’s intent to move the wheelchair, translating the intention into precise control signals to steer and operate the wheelchair.
This provides significant independence and freedom of movement.
Closing Notes
In conclusion, Brain-computer interfaces offer a compelling glimpse into the future of human-computer interaction. While significant challenges remain, ongoing research and development are paving the way for exciting advancements. The potential to improve human lives, enhance capabilities, and address neurological disorders is substantial, and the ethical considerations surrounding this technology will undoubtedly play a critical role in its responsible development and deployment.
FAQ Section
What are the different types of brain signals used by BCIs?
BCIs commonly use signals like EEG (electroencephalography), MEG (magnetoencephalography), and fNIRS (functional near-infrared spectroscopy) to interpret brain activity. Each technique has unique strengths and weaknesses in terms of accuracy, cost, and invasiveness.
What are some ethical concerns regarding BCIs?
Ethical concerns regarding BCIs include potential privacy violations, misuse for human augmentation, and societal implications of widespread adoption. Bias in data used for training BCIs is also a significant concern.
What are the limitations of current BCI technology?
Current limitations include the relatively low accuracy and speed of signal interpretation, the need for significant user training, and the high cost of development and implementation. Furthermore, the long-term effects on the brain are still being researched.
How do BCIs work with neurological disorders?
BCIs show promise in treating neurological disorders by enabling patients to control prosthetic limbs, communicate, or improve motor function through direct brain-computer interaction.